How OOH Object Location Data Influences Opportunity to View

Knowing whether or not your Out-of-Home (OOH) was viewed is integral to understanding how your campaign performed. At Billups, we have three computer vision patents that help us understand whether an OOH unit is in view, whether any objects are blocking the unit, and the unit's orientation. This information helps us establish a viewshed of the vantage points from where the OOH unit can optimally be seen. With mobile location trace data, we can determine how many devices and, likely, eyes were exposed to the unit during its campaign flight.
In this article, you will learn why object location is important, how our technology works, and how you can leverage this information to build better campaigns.
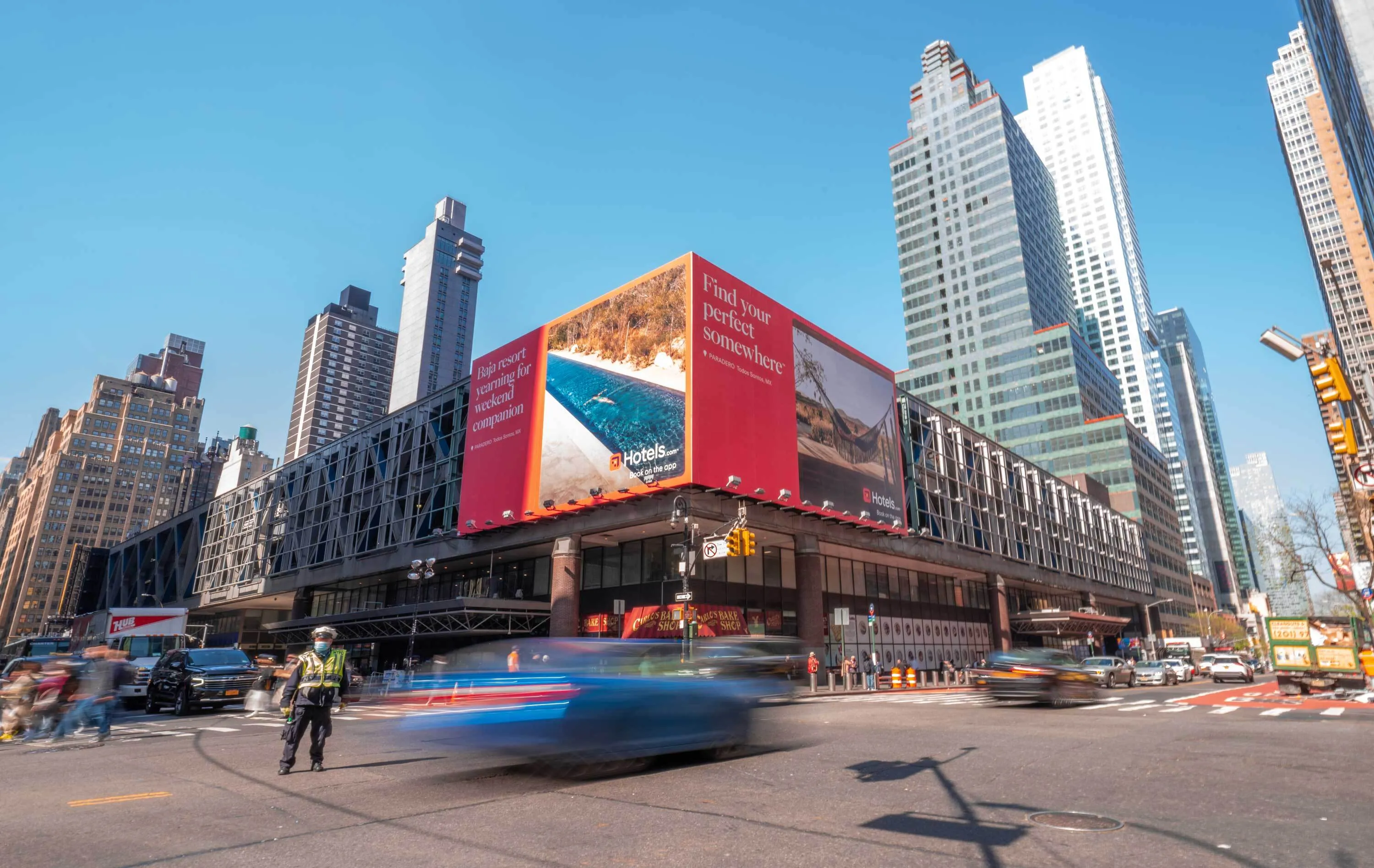
What is Object Location, and How Does it Apply to Out-of-Home?
Many OOH companies will build a viewshed based on a person’s proximity to an OOH unit and determine their Opportunity to See (OTS) simply based on that.
Billups’ patented technology furthers this, factoring in the person’s relationship to the board or Opportunity to View (OTV). Our first computer vision patents are our SSI patents. The patent was named after Shawn Spooner, Billups CTO, and Sicong Chen, Billups Senior Staff Scientist. SSI helps us understand the OOH. Our system can use information from Google Street Level images and other third-party data to determine the format and the surrounding scenery.
By layering images taken about 5 meters apart, our machine-learning models can create three-dimensional images from two-dimensional pictures. Our SSI patent then labels everything in the image to the pixel level.
As you approach the Billboard, taking an image every few seconds, you can get an idea of obstructions. Where are they? What are they? Are there trees? Are they deciduous or coniferous? How visible is the unit? From this object location data, we can determine an accurate viewshed that closely resembles how someone may see an OOH ad.
Knowing object location - of the unit itself, its orientation, and anything else that may be drawing attention away from the OOH or blocking its view- is crucial for evaluating marketing touchpoints.
How Does Object Location Let Us Know What OOH Units Were Viewed?
The SSI patent has a process for mimicking the human visual system. Why is this important? Knowing how the human eyes and brain work together is vital for understanding whether or not an individual viewed an OOH unit.
We think that we see the world in high definition. This is not true. There is a spot at the back of the eye called the fovea. This is the only high-resolution part of your eye. Only a tiny part of our vision is in high definition at any given moment. But our brain continuously examines the environment, giving us the impression that we see everything in our environment clearly.
Our Machine Learning Model is designed to work similarly. We give it images as mentioned above and ask it to recreate what is most important in an image. Which objects are most likely to grab attention in a scene? In what order would someone likely attend to those objects?
We give the model a fixed budget of 1000 bytes to recreate an image. The best way mathematically to do this is to find areas with the most similarity and ignore them. The human eye tends not to notice empty objects like the sky. If there are large pixel areas of sky, the model will encode as much sky as possible in as few bytes as possible. Most of the bytes are spent on what stands out in the scene. What will stand out is dense images with higher intricacy. It is somewhat similar to giving a painter 10 seconds to make a picture from memory. The painter would outline motions first and the most essential objects in the scene. Our model mimics how people process visual information based on attention and eye-tracking studies. It lets you know the top objects that are likely to be seen and the order in which someone would see them.
For example, there is a bridge to Portland with a Wallscape on the right-hand side. It is the most eye-catching object in view. There’s also a Transit Shelter there, but it’s likely to go unnoticed. A person in this environment will most likely see the Wallscape, Bulletin, and the cars around them. The Transit Shelter would be low on the list of priorities.
Once you understand the environment where your ad will be running, you can use it to optimize your board against its background. By changing different design elements, you can make your OOH unit the most eye-catching part of the landscape. In this example, a Transit Shelter will never overpower the Wallscape, but it can be designed to stand out more and get a good number of views at a fraction of the cost.
Physical marketplaces have nuances such as competition for attention, the direction users travel, and objects blocking part or all of the unit up until a certain point. Our Object Location Model ensures that we can evaluate inventory and exposures in the same fashion a user in the marketplace would. Based on the information gathered using our SSI and Object Location Facing patents, we can determine the optimal viewshed for an OOH unit by knowing what else is in the scenery, the orientation of the unit, and the direction of traffic.
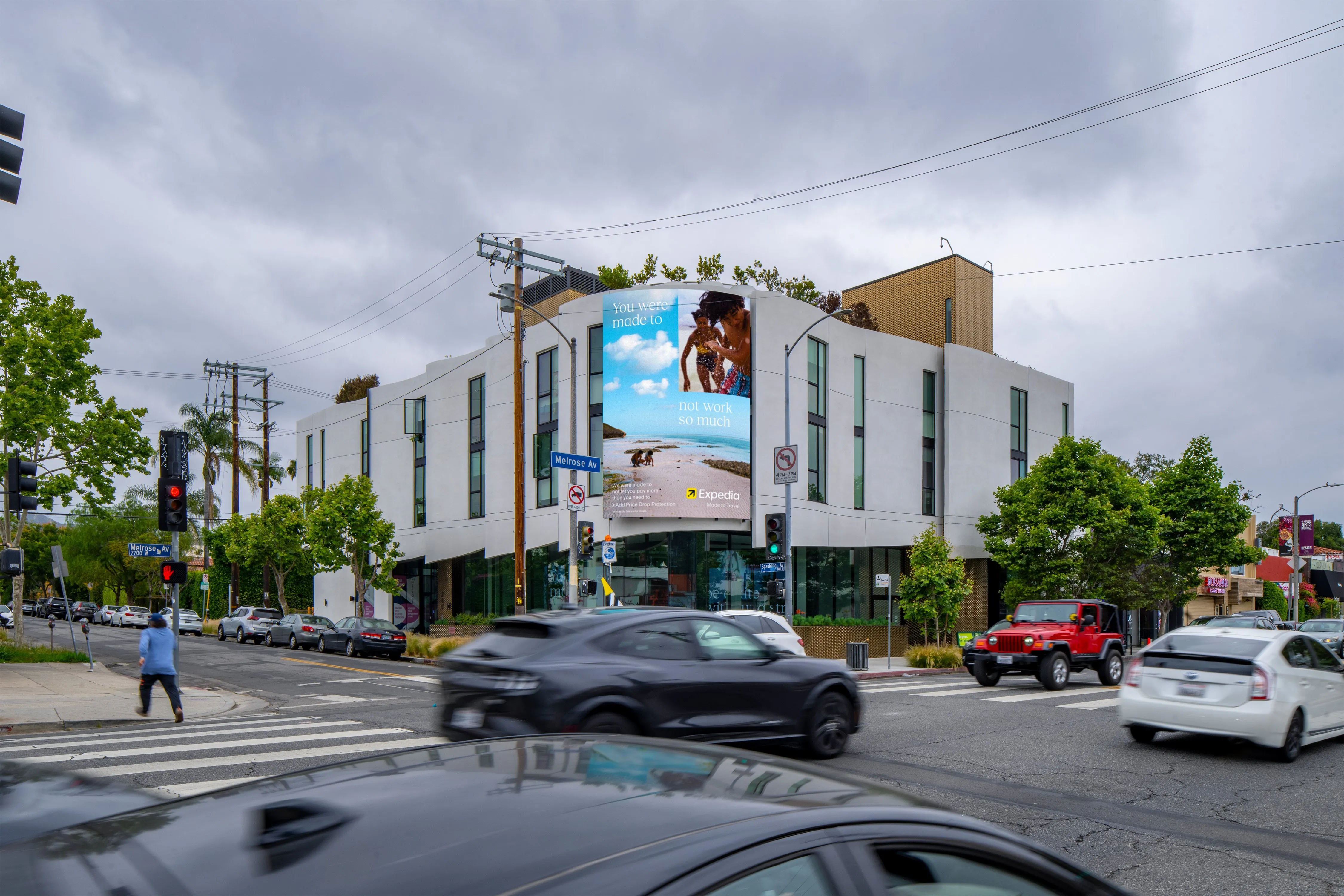
How Does Object Location OOH Scoring Work?
Once we have determined the viewshed for an OOH unit and how likely it will be noticed in a scene, we can score that unit against its surrounding environment. Using mobile device data, we can see how many devices have traveled through that viewshed. We calculate the probability of viewing the unit, and how much attention would be paid to the unit to determine whether or not a user was likely exposed to the advertising based on the aforementioned factors.
Mobile devices send out location traces at different times and rates. Most traces will be sent from places people frequent. Most people have two to three places they frequent: work, home, and a third place. By reviewing a user’s history of mobile traces and where they send the most signals from, you can determine the primary areas they tend to go to. Knowing where they tend to go and traces along the paths connecting those locations, you can get a pretty good idea of the route they likely took to get there. We use this location data to reconstruct their probable journeys and any OOH they may have encountered, whether or not their journey has passed through the viewshed itself.
Audience exposures predict how many users had an optimal opportunity to view an OOH campaign or the Opportunity to Experience (OTE) that campaign. Given the location of an OOH unit and an individual device’s daily journey, we can judge the likelihood of an exposure to the unit. This likelihood measures the success rate of the specific advertisement capturing that person’s attention. We score exposures based on the mobile device data and factor in criteria based on the human visual processing system, including the speed users are traveling, the direction, elevation, and any competition for attention.
Audience exposures apply machine learning algorithms to make precise, accurate, and stable predictions of audience view counts. Knowing who was exposed to your campaign can help empower and contextualize your OOH campaigns.
Find the Best Placements for Your Campaign with Billups
Our patented object location and scoring technology validate who was or was not exposed to OOH. Billups Analytics™ puts the power in the advertiser's hands so that they can know how many people were exposed to their OOH units. Data breakdowns include delivery by unit, audience demographics, and campaign reach and frequency metrics. Using Billups Analytics, you can track campaign performance in near real-time and adjust your campaign for optimal performance. Contact us to learn more today!
Helpful resources
Explore our article library